Excel - Champions League
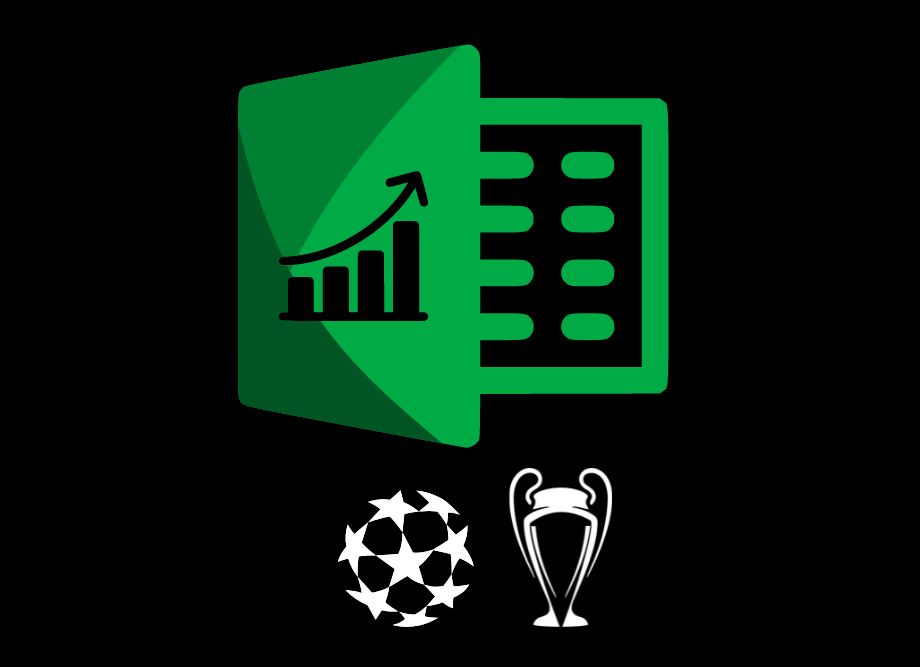
Immerse yourself in the exhilarating world of Champions League analytics using Microsoft Excel.
This micro course is tailored for enthusiasts who want to master data manipulation and visualization with real-time datasets from the Champions League.
Learn to clean, analyze, and visualize player statistics, team performance, and match trends.
Gain the skills to create dynamic dashboards that offer valueable insights.
This micro course is tailored for enthusiasts who want to master data manipulation and visualization with real-time datasets from the Champions League.
Learn to clean, analyze, and visualize player statistics, team performance, and match trends.
Gain the skills to create dynamic dashboards that offer valueable insights.
Excel Bootcamp
Champions League Data Lab
The Intro course is free
-
Video Introduction
-
Paid Section Preview
-
Theory
-
Case Study Preview
-
Practice
#1 Excel Bootcamp
Sections included :
-
Working with tables
-
CountA() Function
-
CountIFS() Function
-
Exploring Excel
-
CountIF() Function
-
SumIF() Function
-
IF() Function
-
Data Validation & CountIF()
-
SumIFS() Function
-
Average() Function
-
AverageIF() Function
-
AverageIFS() Function
-
Pivot Tables
#2 Advanced Excel
Sections included :
-
VLOOKUP() Function
-
MATCH() Function
-
LEFT() Function
-
HLOOKUP() Function
-
TRIM() Function
-
RIGHT() Function
-
INDEX() Function
-
CLEAN() Function
-
MID() Function
-
SUBSTITUTE() Function
-
Data Cleaning
#3 Playground
Sections included :
-
Case Study 1: The Home Ground Advantage
-
Case Study 2: Clash of the Titans
-
Case Study 3: Win - Loss Ration
-
Case Study 4: Analyzing Data based on Performance Ranking
#4 Practice Section
Sections included :
-
IF() Function Exercises
-
COUNTA(), COUNTIF() & COUNTIFS()
-
SUM(), SUMIF() & SUMIFS() Functions
-
AVERAGE(), AVERAGEIF() & IFS()
Data Labs
#5 Case Studies
A Data Lab is essentially a dynamic learning environment where we put into practice the latest skills we've acquired in Excel through the League of your choosing.
In this section, our focus shifts to the realm of Champions League data.
Data Lab serves as a practical course where we immerse ourselves in hands-on Case Studies, applying our knowledge to gain insights and make data-driven decisions.
With each Case Study, we explore new dimensions of data analysis, allowing us to delve into the latest trends and uncover hidden patterns within the world of Champions League data.
In this section, our focus shifts to the realm of Champions League data.
Data Lab serves as a practical course where we immerse ourselves in hands-on Case Studies, applying our knowledge to gain insights and make data-driven decisions.
With each Case Study, we explore new dimensions of data analysis, allowing us to delve into the latest trends and uncover hidden patterns within the world of Champions League data.
Sections included :
Case Study #1 : Internazionale's Season Performance
Internazionale ⚽ showed a solid defensive performance 🛡️, with some variability in offensive performance 🎯 and possession 🎛️. The team seems to have efficiently utilized their chances 🏁, showing an ability to win matches 🏆 even when not dominating possession ⏳. As with any data analysis 📊, it's important to consider the context and limitations ⚠️ of the data, and to interpret the findings 🔍 accordingly.
Case Study #2 : Napoli's Season Performance
Overall, based on the dataset, Napoli demonstrated an impressive performance in the 2022/23 Champions League season ⚽. Their high-scoring, possession-based approach, balanced with a proactive and solid defense 🛡️, appears to be effective, as reflected by their substantial goal difference 📈. The consistency in their goal-scoring ability 🥅, coupled with their defensive prowess 💪, suggests a well-rounded team capable of competing at the highest level 🌟.
Case Study #3 : Celtics vs RB Leipzig
Based on the comprehensive analysis of the match data, RB Leipzig displayed a superior performance 🏆 in the match against Celtic. Key factors leading to this conclusion include Leipzig's higher number of accurate passes ⚽, successful final third entries 🎯, shots on target 🥅, superior player involvement 👥, and fewer fouls committed 🚫. Leipzig's superior tactical discipline 📋, fitness management 💪, and effective utilization of substitutes 🔁 also played a crucial role in their performance. This case study underscores the power of soccer analytics 📊 in providing in-depth insights into team performance and strategic decision-making 💡.
Case Study #4 : Juventus's Season Performance
🎯 Juventus demonstrated some serious strengths and knack for creating big chances during the 2022/23 Champions League season. 😅 Yet, they found themselves grappling with defensive consistency, as goals were conceded in every match. 🥅
🎢 Their performance fluctuated greatly across matches, sometimes showcasing high scoring and passing accuracy 🥅⚽️, while experiencing difficulties in others. Although they fashioned a respectable number of scoring chances, their goal conversion rate wasn't always on point. 🎯 There were games where high accurate passes ⚽️➡️ didn't translate into corresponding high goal numbers. ⚽️🚫
📊 In matches where Juventus were on a roll, creating more significant opportunities (more than two big chances 🎯🎯), their conversion rate was impressive, with an average of three goals per game. ⚽️⚽️⚽️ This suggests that the secret sauce 🗝️ to their offensive success could lie in generating clear-cut chances.
💪 On the defensive side, despite racking up a substantial number of interceptions, the team fell short of keeping a clean sheet in any of their matches. 🥅🚫 The data insinuates that these interceptions didn't lead to defensive success, pointing to possible hiccups with their defensive strategies or execution. 🤔💭
🔎 In summary, Juventus showed promise in the 2022/23 Champions League season, including their passing 🤝 and chance creation 🎯. However, they also ran into obstacles, notably in converting possession into goals ⚽️ and maintaining a staunch defence 🛡️. Future success may involve ironing out these issues, while further capitalizing on their strengths.
Case Study #5 : Milan's Season Performance
The 2022/23 Champions League season seems to have been a slam dunk for Milan 🎉, flaunting consistent performances in both defensive 🛡️ and offensive ⚽ aspects of the game.
Their style of play 🎮 showcased versatility, nimbly adapting to the unique circumstances of each match. They kept a healthy balance of possession ⚖️⚽, converting opportunities into big chances 🎯, which resulted in a considerable number of goals 🥅.
Despite some rollercoaster ride in performance metrics 📊, the team displayed tactical adaptability 🌐 and resilience 💪 across the tournament. They remained steadfast, proving that they can weather any storm and rise to the occasion. 🌪️
Case Study #6 : Bayern Munich vs Paris Saint-Germain
The match between Bayern Munich and Paris Saint-Germain (PSG) is a detailed study in contrasting styles and outcomes. From a broad perspective, Bayern Munich emerged as the victor with a 2-0 scoreline ⚽, despite having a lower average number of accurate passes per player (19.70) compared to PSG (25.36). One could argue that Bayern Munich were more efficient with their passing, converting fewer passes into more goals.
The average time played by Bayern's players was slightly lower (43 minutes) ⏱️ than that of PSG (45 minutes). This difference could be attributed to Bayern's substitutions strategy which might have focused on maintaining a fresh team and tactical adjustments.
The statistics further indicate that Bayern Munich created two big chances 🎯 compared to none by PSG. This statistic, coupled with Bayern's two goals, suggests that Bayern was able to translate their opportunities into tangible results more effectively.
The number of times players from each team were dispossessed was comparable, with Bayern slightly higher (0.52 times) than PSG (0.45 times). This suggests a highly competitive match in which both teams pressured the opposition effectively. Aerial duels were also closely contested, with PSG winning slightly more (12) than Bayern Munich (10).
A deep dive into the match statistics by player positions reveals more nuances. Among Bayern Munich's squad, the attacking midfielders were instrumental, contributing substantially to total passes, total crosses, and total contests. They also demonstrated their defensive prowess, notably in winning possession in all thirds of the pitch.
Defenders also played a vital role for Bayern, making a significant contribution to total clearances and long balls. For PSG, midfielders were responsible for a significant number of passes and contests, but their efforts did not translate into goals.
In terms of ball possession, both teams lost control a similar number of times, with Bayern losing it 133 times and PSG 137 times. However, Bayern slightly outperformed PSG in terms of possession won in each third of the pitch.
The statistics on passing accuracy reveal that Bayern Munich had fewer accurate passes than PSG. However, the type of passes where Bayern outperformed PSG—back zone passes, chipped passes, crosses, and long balls—suggests a strategy that focused on maintaining possession, bypassing the midfield, and rapidly advancing the ball into scoring positions.
In summary, Bayern Munich's victory over PSG can be attributed to their strategic use of passes, efficient conversion of chances into goals, and effective possession winning tactics across the pitch. Despite PSG's superior passing statistics, they were unable to capitalize on these opportunities, resulting in a scoreless match.
Sports Analytics School powered by Sportrated
Copyright © 2023